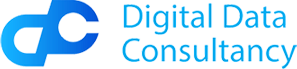
A report recently published by IDC predicts that the collective sum of the world’s data will grow from 33 zettabytes this year to a 175ZB by 2025, for a compounded annual growth rate of 61 percent.
The tools, technologies and concepts to create, collate and manage these volumes of data are also evolving at the same rate.
Most organizations have invested or are planning to invest in resources to manage this data. Yet many are finding that with their existing traditional data management approach, the sheer amount of data is far too challenging to manage. The cost of managing this data has gone up but the return of these investments is steadily decreasing. This is forcing enterprises to rethink their traditional data management strategy.
What has transpired is that though these organizations have invested in cloud, big data and analytics, with a traditional data management approach they often find themselves ending up with siloed stacks unable to meet the growing business demands of real time data delivery, self-service data plat formats etc. to name a few. This is where these organizations need to adapt to the concepts of Modern Data Management.
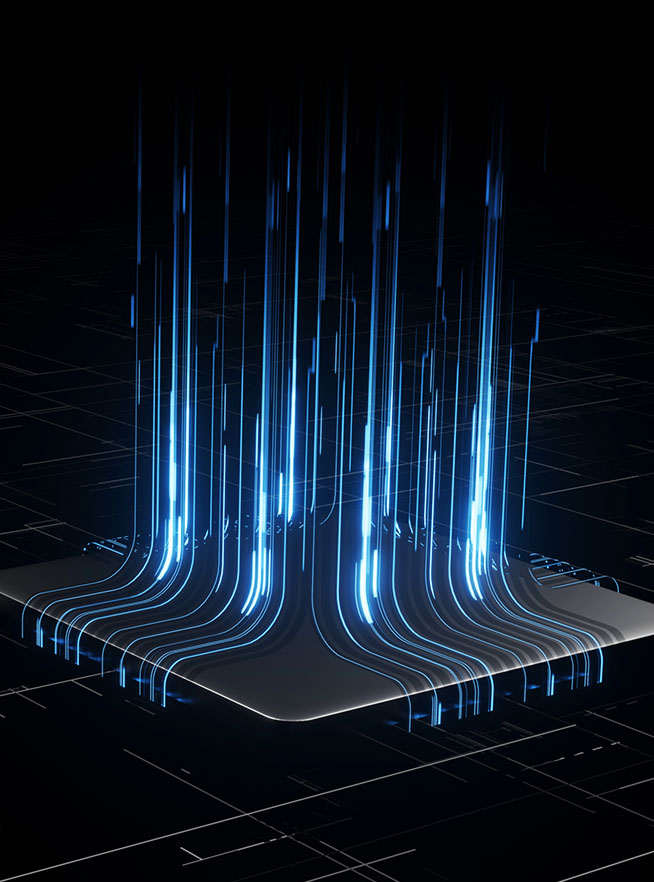
So, what is Modern Data Management?
Modern Data Management is a combination of approaches and capabilities
to ingest, model, manage and overn data to make it accessible to all levels
of stakeholders of an organization in an incredibly agile way.
This is a very broad-brush definition but that is the nature of this subject. It is broad and complex, but very necessary for organizations to get it right sooner than later. It is only going to get more and more complicated as organizations delay getting this in place.
What this article hopes to do is to scratch the surface of this complex topic by focussing on few key components needed to be in considered for a successful Modern Data Management strategy. They are as below:
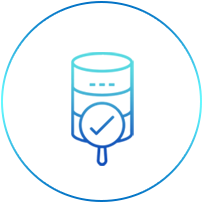
Data Quality
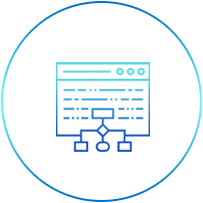
Data Architecture
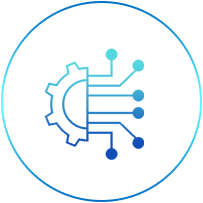
Data Ingestion
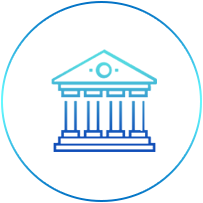
Data Governance
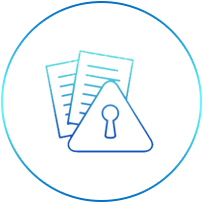
Data Compliance &
Protection
Data Architecture
Most of the organization would have built their data architecture foundations based on tradition concepts like relational databases, data warehousing, ETL etc. Then came along the developments in Big Data, NoSQL etc. These capabilities, in most cases, have been added on to or patched into the existing architecture making it fragile. Over a period, these data warehouses and data lakes have evolved to become siloed stores making it difficult to join them up.
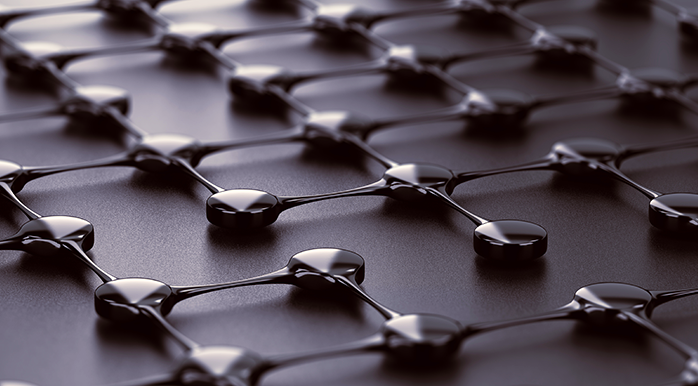
Leaders and experts in the fields of data architecture need to visualize a Data Core build on combination of traditional concepts like MDM, Data Warehouse, ODS etc and modern concepts like cloud, NoSQL, sandboxes etc. This Data Core built with consumer of data as the focus and it must house profiled, curated and trustworthy data for analytics and BI purposes.
In the end, a modern data management architecture does not need to replace services, data or functionality that works well internally as part of a vendor or legacy application. Instead, it is optimized for consumption and sharing data across
Data Governance
As the volume of data increases, so do the responsibilities of data governance. Data governance now needs to be more self-serving, agile and fast.
- They need to have a strategy to govern big data. This is challenging because big data is so diverse, some of it is coming from outside the organization and it is generally complex.
- They need to take advantage of data discovery and data governance tools
- With Cloud becoming strategy for most of the organization. Data Governance extend or modify their policies and accountabilities to include data the resides in Cloud storages that can be private or public.
- Focus on value produced, not just methodology and processes.
- Have Data stewards as problem solvers and have them included in projects.
Finally, Data Governance should become more agile and find a right balance between old-style and new-world governance practices. It needs to evolve from a mind set of authority and control to as-a-service.
Data Quality Management
Organizations are dealing with more and more big data which involves working with unstructured data. The data quality practices and techniques that we use for traditional structured data don’t work well for big data. In the traditional data quality world, we are geared toward cleansing data to improve content correctness and structural integrity. In the big data world, quality is more elusive. Correctness is difficult to determine when using data from external sources, and structural integrity can be difficult to test with unstructured and differently structured (non-relational) data.
With big data, quality must be evaluated based on the use case for which the data is being used for. As with analytics, the need for data quality can vary widely by use case. The quality of data used for financial consumption, for example, may demand a higher level of accuracy than data used for customer demographics.
The approach and framework for data quality in today’s world should be flexible to cater to the use case data quality KPI requirements.
Data Ingestion
We don’t have to go too long into the past to realise that most of the data generated for BI and analytics was generated within an organization. Things have change and changed fast. There is an increase in share of data coming into the organisation with the invent of bigdata and social media data. Also, business roles with a drive to get more insights from new data sources are increasingly becoming interested in data ingestion capabilities which in the past was considered for more tech-savvy segment of the organization.
Modern Ingestion capabilities should at least tick-off
- Being a hybrid to converge data from both traditional and cloud-based applications.
- Being agile, flexible and elastic with ability to support any kind of data integration & migration process - anytime, anywhere
- Having high degree of reusability. As for technical teams, reusable services speed up the creation of more complex data integration processes
- Having processes and tools to continuously handle data twists and turns over time
Data Compliance & Protection
Data is no longer restricted within four walls of an organization. Systems and applications are becoming increasingly open and interconnected. With data growing at an explosive rate, modern businesses need ways to lower storage and operational costs and at the same time stay compliant to data protection regulations like GDPR to avoid hefty fines.
Organization need to look at data protection solutions that offer high performance, lower storage and operating costs, and inherent data security. They need to consider a holistic platform that offers ease of deployment, ease of management, storage efficiency, and the flexibility to leverage a multi-cloud environment.
01our trends
News & Papers
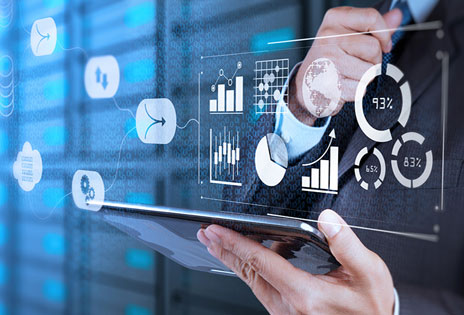
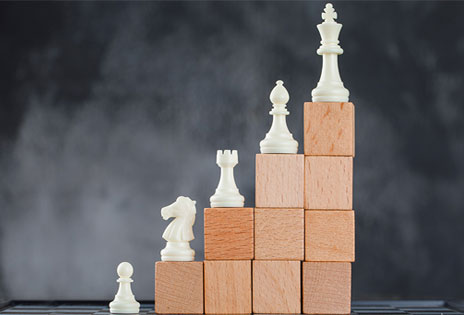
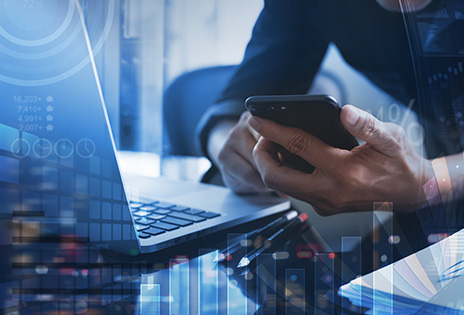
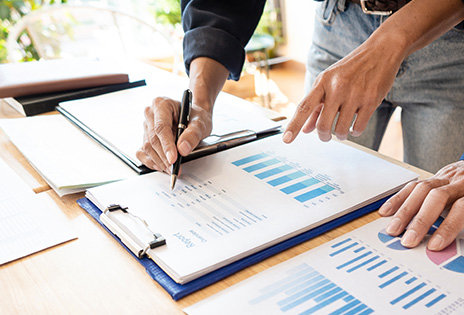